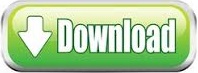

- #Download matlab 2018b deep learning how to
- #Download matlab 2018b deep learning full
- #Download matlab 2018b deep learning code
- #Download matlab 2018b deep learning download
How to integrate the RGB- uv histogram block into my network?įor Matlab 2018b and 2019a, please check examples given in RGBuvHistBlock/add_RGB_uv_hist.m.
#Download matlab 2018b deep learning full
In the paper, we used the full camera pipeline in A Software Platform for Manipulating the Camera Imaging Pipeline, ECCV'16 to render images to the sRGB space with our estimated illuminant vector. In the given demo, we show raw-RGB images after white balancing and scaling it up by a constant factor to aid visualization.

Why does the demo show faint colors compared to what is shown in the paper? You can also use all trained models and report the averaged illuminant vectors for evaluation (i.e., ensemble model).
#Download matlab 2018b deep learning code
An example code to evaluate testing image set is provided in evaluate_images.m for Matlab. You can report results of one model or the best, mean, and worst results obtained by all models. Then, you can use any of our trained models for testing. The input images should be stored as uint16 PNG files after the normalization. How to report results of the trained models using a new set of raw-RGB images?įirst, be sure that all testing images are in the raw-RGB linear space and the black level/saturation normalization is correctly applied. Our method, however, targets to work independently from the camera sensor. Our work is proposed to reduce the differences between camera sensor responses (that is why mostly all of learning-based illuminant estimation models are sensor-dependent and cannot generalize well for new camera sensors - see On Finding Gray Pixels, CVPR'19 for interesting experiments that highlight this point). To corret your sRGB-rendered images, you can check When Color Constancy Goes Wrong:Ĭorrecting Improperly White-Balanced Images, CVPR'19 for white balancing sRGB-rendered images (an online demo is provided).Ĭan I test images captured by camera sensors different than the camera sensors used for training (i.e., NUS, Gehler-Shi, and Cube/Cube+ datasets)?
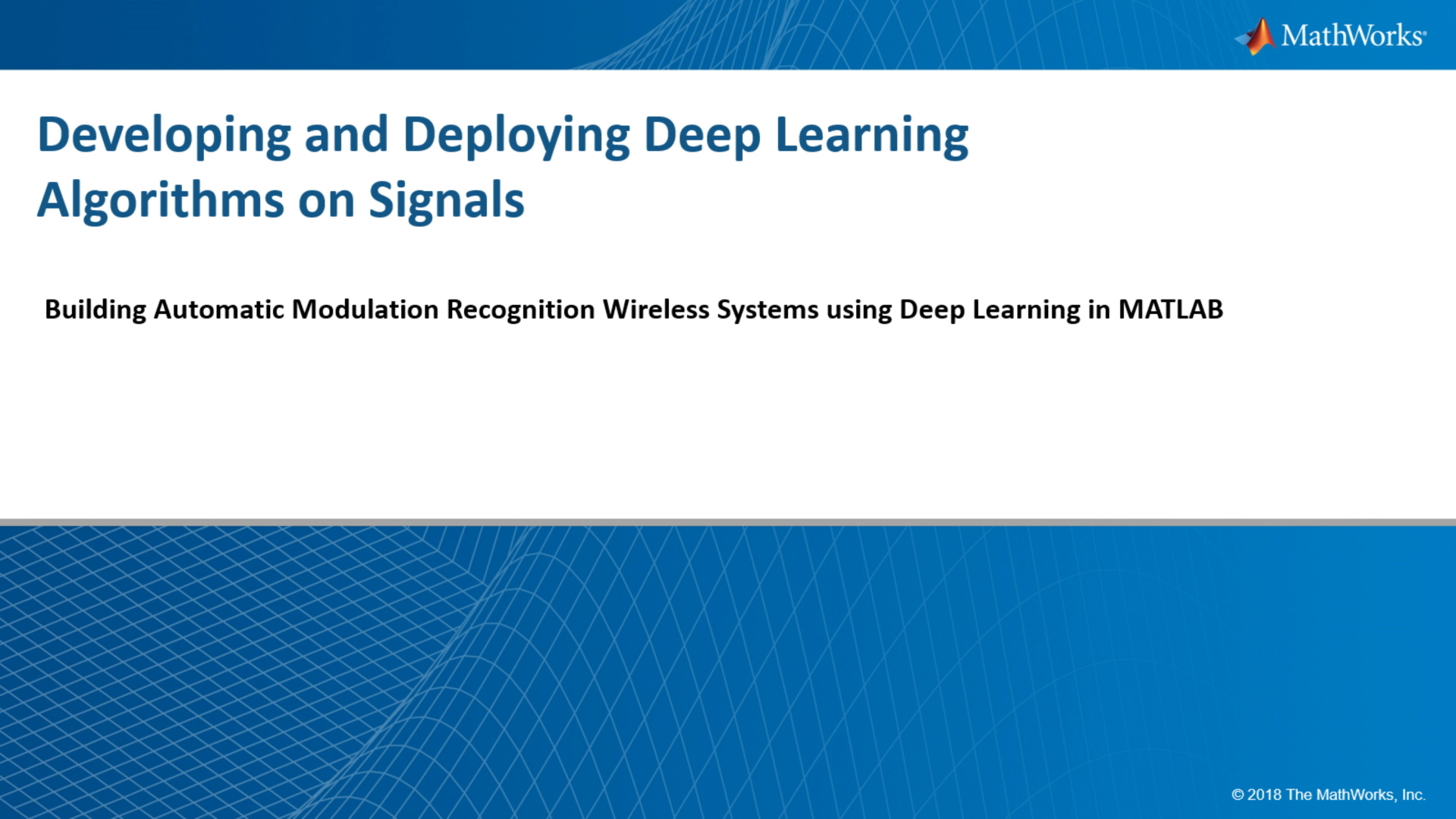
Our method works with linear raw-RGB images, not camera-rendered images. FAQ Can I use it to correct sRGB-rendered JPEG images? This is very important since all trained networks expect to get uint16 input images after applying the black/saturation level normalization. The input image file must contain the image raw-RGB values after applying the black/saturation level normalization. Each model is named based on the validation set used during the training (for example, the model trained_model_wo_CUBE+_CanonEOS550D.mat was trained using all raw-RGB linear images from NUS and Gehler-Shi datasets without including any example from the CanonEOS550D camera in Cube/Cube+ datasets). Each model was trained using different camera sensors, as discussed in our paper. You can test any of our trained models located in models directory. You can change the model_name and image_name variables to choose between our trained models and to change input image filename, respectively. The supported versions are: Matlab 2018b, Matlab 2019a, or higher. In demo.m, you should select the version of Matlab by changing the value of Matlab_ver. Run install_.m, then run demo.m to test our trained models. The provided code for Matlab 2019b or higher gives almost the same results. The original experiments were done using Matlab 2018b. Deep Learning Toolbox for Matlab 2018b or higher.Provide a dataset by replacing the file data.Sensor-Independent Illumination Estimation for DNN ModelsġYork University 2Samsung AI Center (SAIC) - Toronto
#Download matlab 2018b deep learning download
ATL_MatlabĪTL: Autonomous Knowledge Transfer From Many Streaming ProcessesĬlone ATL_Matlab git to your computer, or just download the files. Update: You can find ATL_Python already by following this link. This will make it easier for researchers to use ATL in their benchmarks. In the following weeks, we will upload a version of this code on Python (Pytorch, with autograd enabled and GPU support). = ,ĪTL_Matlab uses Matlab and it was the original code used for this paper.
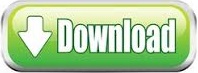